Together, We Succeed.
Data analytics and decision making in business
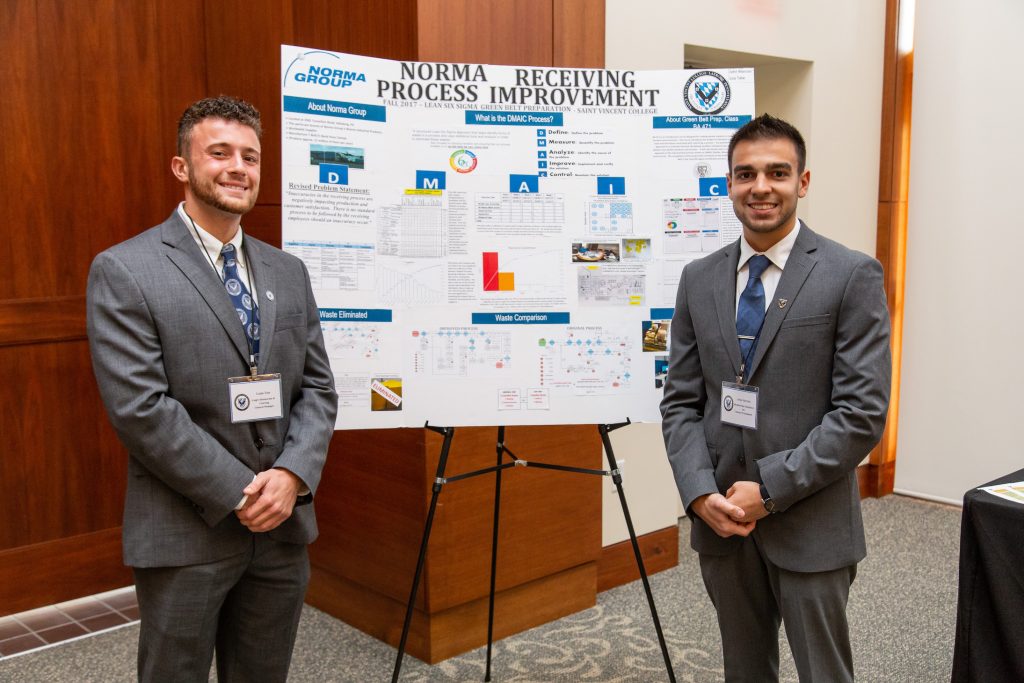
When it comes down to it, the main purpose of data analytics is to help companies, non-profits, and other organizations and businesses make better-informed decisions. The ways that data analytics are implemented into decision-making are growing exponentially. Below are a few examples of ways decisions are made using data analytics and how Saint Vincent College business data analytics students are implementing them in their own coursework.
In a previous post, we discussed various stages in a data science project and the methods and tools implemented in each. Four key types of analytics are used to make decisions at these various stages, each one building on the previous. They include:
- Descriptive analytics is the foundational raw data that describes what happened or what is currently going on.
- Diagnostic analytics seeks to understand why the data occurred/why it happened;
- Predictive analytics uses this past data and understanding of why it happened to predict future trends or events; and
- Prescriptive analytics brings all of this together to make the big decision: What should we do next?
Each of these carries the opportunity to inform decision-making. For example, descriptive analytics in real estate sales data might show that more homes are sold in a specific area during the summer months. This data can easily be visualized and used by both home buyers and sellers to make a decision around when it might be best for them to sell or buy.
Diagnostic analytics might take this example a step further and use other data, such as the housing market in general, data surrounding schools in that specific area, or the demographics of the people moving to that area, and more. This might help an analyst understand that perhaps more families are going to that area, and families are more likely to move their school-aged kids during summer break so they wouldn’t have to start at a new school mid-year.
Keeping with this same example, predictive analytics would help real estate professionals to use this very predictable information of a hotter housing market in the summer to make decisions around their clients’ own home sale. Prescriptive analytics could potentially help professionals use this data to test scenarios and implement the best course of action (i.e., is it better to sell a home in the summer when there are many buyers and sellers or in the winter when there is low supply?).
Data analytics can improve decision-making by identifying consumer patterns, utilizing data to drive performance, and by mitigating risk. These can include understanding customer behavioral patterns, implementing sales forecasts, cost-efficiency analysis, and price optimization.
It would seem logical that the more data implemented and the more factors at work, then the more sound decision-making. However, the key is understanding potential false correlations, failure to bring in a full picture, and even potential prejudices. Having a full understanding of how data can and should be utilized, including data ethics, is the reason for having people who can reconcile the risks of using strictly machine and artificial intelligence data tools.
At the Kennametal Center for Operational Excellence at Saint Vincent, business students take advantage of internship opportunities across a wide variety of industries and disciplines, including management, healthcare, manufacturing, operations, sales, and supply chain, gaining real experience helping companies and organizations make these types of informed decisions.